RAG (Retrieval-Augmented Generation)
: Query가 주어졌을 때, 데이터베이스에서 관련된 정보를 검색하여 프롬프트에 contxt를 추가.
- 장점
- Hallucination 최소화
- Fine-tuning 대비 저렴한 비용으로 관련 정보 검색 가능
- 한계
- 전체 corpus에 대한 추상적 요약이 어려움
- Context window 한계로 인한 정보 손실 (”lost in the middle” 현상)
- Graph RAG (GraphDB + RAG)
- 데이터를 지식 그래프 구조로 변환 → 그래프 검색을 활용한 RAG
Knowledge graph 지식 그래프
:Knowledges의 구조화된 표현
- Node: entities or concepts
- Edge: relationships between entities
Microsoft GraphRAG
In contrast with related work that exploits the structured retrieval and traversal affordances of graph indexes, we focus on a previously unexplored quality of graphs in this context: their inherent modularity (Newman, 2006) and the ability of community detection algorithms to partition graphs into modular communities of closely-related nodes (e.g., Louvain, Blondel et al., 2008; Leiden, Traag et al., 2019).
- 핵심: 데이터를 그래프로 표현, community detection 알고리즘 활용
- 목표: global과 local 관점의 효과적인 context 추출
GraphRAG Pipeline
Indexing Time
- Text extraction and chunking
- Chunk 내 Entity 추출 (name, type, description 중심)
- domain-tailored summarization: 특정 카테고리에 특화된 element를 추출
- claims: element(node) 간 공변성(covariate)을 정보로 담기 위해 subject, object, type, 시작/끝 날짜 등 추가 정보를 추출
- Element summary 생성
- Abstractive summarization을 개별 그래프 요소에 대한 정보 블록으로 변환
- Community detection (Leiden 알고리즘 사용)
- hierarchical community structure 형성
- 각각의 노드들은 mutually-exclusive, collective-exhaustive 형태의 정보를 가짐
- 직접적인 연결이 없는 노드들이더라도, 간접적인 연관이 있을 법한 (유사한) 노드들끼리 같은 community에 속하게 된다.
- Community summary 생성
- Leaf-level communities
- 우선순위에 따라 Element summary들을 LLM context window에 추가
- 각 community edge를 소스와 타겟 노드의 중요도에 따라 내림차순으로 정렬
- 가장 중요한 엣지부터 해당 엣지의 소스/타겟 코드, 연결된 공변량 및 엣지 자체의 설명을 LLM context window에 추가
- 우선순위에 따라 Element summary들을 LLM context window에 추가
- Root-level communities
- (token limit 이내) Leaf-level과 동일
- (token limit 초과) 관련 element summary들을 sub-community summaries로 반복적으로 대체
- Leaf-level communities
Query Time
- Hierarchical community structure ⇒ 다양한 level의 community summaries를 활용해 질문에 답변할 수 있다!
- Community summaries 기반으로 Global answer 생성
- Prepare community summaries
- community summaries 셔플 + chunking: 관련 정보가 한 번에 집중되지 않도록 분산시키기 위함
- Map community answers
- Community answer 생성 (병렬): Chunk 당 하나의 intermediate answer 생성
- Helpfulness score (0-100)
- Reduce to global answers
- Intermediate answers H-score 기준 내림차순 정렬 → (토큰 제한에 도달할 때까지) 순서대로 새로운 context window에 추가
- 최종 context 기반으로 Global answer 생성 및 반환
- Prepare community summaries
평가
평가용 질문 생성과 답변 평가 둘 다에 LLM 사용
평가 방법
데이터셋
- Podcast transcripts, News articles
- 600-token text chunks, with 100-token overlaps between chunks
쿼리 생성
- Global sensemaking tasks에 대해서 RAG 시스템의 효율성 평가
- → 데이터셋 내용에 대한 전체적인 이해를 요구하는 질문이 필요하다.
- Activity-centered approach(활동 중심 접근법)을 통한 질문 생성 자동화
| N = 5 (데이터셋 당 125개 테스트 질문 생성)
1. 데이터셋에 대한 짧은 설명 제공
2. (LLM에) N명의 잠재적 사용자, 사용자 당 N개 tasks 식별 요청
3. (LLM에) 각 (user, task) 조합에 대해 전체 corpus 이해가 필요한 N개 질문 생성 요청
비교 조건(6가지)
- Graph community levels: C0(root), C1(high), C2(intermediate), C3(low)
- TS: Text summarization method (map-reduce approach)
- SS: Naive “semantic search” RAG
평가 지표
- LLM as a judge: head-to-head comparison approach using an LLM evaluator
- 측정 요소
- 포괄성 (Comprehensiveness): 답변이 질문의 모든 측면과 세부 사항을 얼마나 잘 다루는가?
- 다양성 (Diversity): 답변이 다양한 관점과 통찰을 얼마나 풍부하게 제공하는가?
- 이해력 (Empowerment): 답변이 독자가 주제를 이해하고 판단을 내리는 데 얼마나 도움이 되는가?
- 직접성 (Directness): 답변이 질문에 얼마나 명확하고 구체적으로 답하는가?
실험 구성
- Fixed context window size: 8k tokens
실험 결과
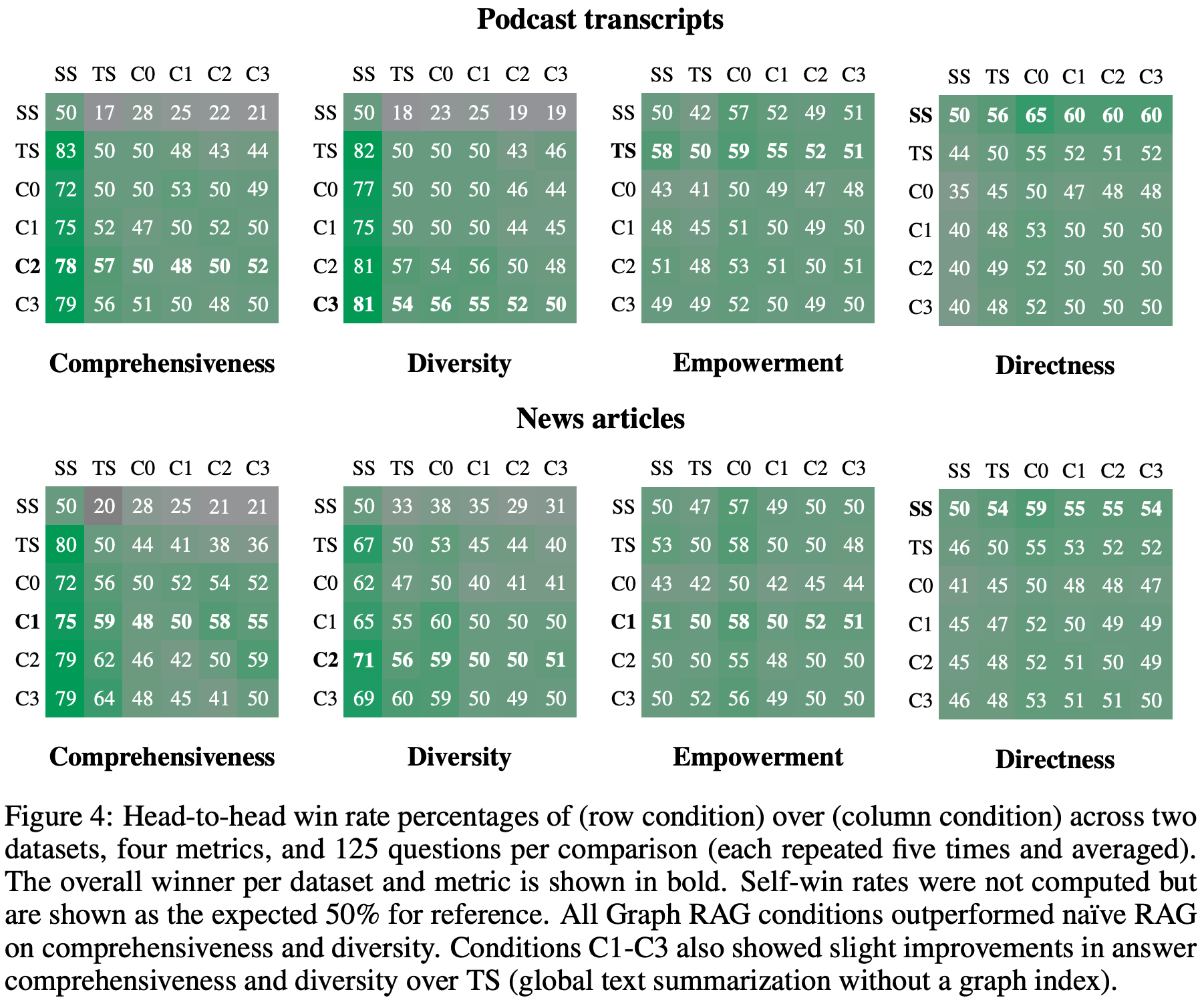
- Global approach vs. Naive RAG: Comprehensiveness, Diversity 측면에서 모든 GraphRAG가 naive RAG 보다 나은 성과.
- Community summaries vs. Source texts: Comprehensiveness, Diversity 측면에서 C1-C3이 TS (Text Summarization) 대비 약간의 개선을 보임.
- Empowerment: 구체적인 예시, 인용구, 출처가 있는 답변이 더 높은 평가.
관련 연구
관련 연구와의 차별점
- Self-generated graph index 사용
- 글로벌 요약을 위해 데이터를 분할하는 데 그래프의 modularity를 활용
관련 연구: Graphs and LLMs
Knowledge Graph Creation and Completion
- LLM을 사용한 지식 그래프 생성 및 완성
- 인과 그래프(Causal Graph) 생성
Advanced RAG with Graphs
- Knowledge Graph Index: 그래프 인덱스를 사용하는 RAG 시스템(KAPING), 서브그래프 구조 검색 시스템(G-Retriever)
- Graph Metrics: 그래프 메트릭스를 사용하여 질의를 수행하는 시스템(Graph-ToolFormer)
- Fact-grounded Narrativeㄴ: 검색된 서브그래프의 사실을 기반으로 서사를 생성하는 시스템(SURGE), 내러티브 템플릿을 사용하는 시스템(FABULA)
Graph Databases: LangChain, LlamaIndex 등
Graph-based RAG 응용
- NebulaGraph
- LLM을 NebulaGraphDB에 통합
- Neo4j
- NaLLM: Neo4j 그래프 DB와 LLM 통합
- LLM Graph Builder: 비정형 데이터로부터 자동으로 knowledge graph 구축
결론
특성 | 기존 RAG | GraphRAG |
데이터 구조 | 평면적 문서 | 그래프 기반 |
검색 방식 | 키워드/의미 검색 | 커뮤니티 기반 검색 |
컨텍스트 범위 | 지역적 | 전역적 + 지역적 |
요약 능력 | 제한적 | 계층적 요약 가능 |
장점 | 직접적인 질문(예: “X에 대해 알려줘”)에 대한 답변에 우수 | 전체 텍스트에 대한 광범위한 이해가 필요한 복잡한 쿼리에 더 정확한 응답 생성 |
적합한 사용 시나리오 | 키워드 검색, 의미 검색이 필요한 상황 | 글로벌 context 이해, 다양한 관점 제시가 필요한 상황 |
- 글로벌 접근 방식: Graph RAG는 지식 그래프 생성, 검색 보강 생성(RAG), 질의 중심 요약(QFS)을 결합하여 전체 텍스트 코퍼스에 대한 인간의 이해를 지원하는 글로벌 접근 방식을 제시.
- 성능 향상: 초기 평가에서 Graph RAG는 포괄성 및 다양성 측면에서 나이브 RAG 대비 상당한 성능 향상을 보여주었으며, 그래프 없는 글로벌 텍스트 요약 방법과 비교하여도 유리한 비교 결과를 나타냄.
- 효율성 (효율적인 글로벌 쿼리 처리): 여러 글로벌 질의가 필요한 상황에서, 루트 수준 커뮤니티 요약을 사용한 GraphRAG는 나이브 RAG보다 우수하며, 다른 글로벌 방법과 비교해도 낮은 토큰 비용으로 경쟁력 있는 성능을 제공.
추가 정보
Query Time
: Local Search와 Global Search (위의 GraphRAG Pipeline > Query Time 참고)
- 미시적/ 거시적 질문에 따라 선택(Routing)하여 답변하기 위해
Local Search
: Entity-based Reasoning
- 지식그래프의 정형 데이터 + 입력 문서의 비정형 데이터 결합
- Query time) 관련 엔티티 정보로 LLM context 증강
- 입력 문서의 특정 entity에 대한 이해가 필요한 질문에 답변할 때 적합하다.
- 예: What are the healing properties of chamomile?
참조
- (논문) From Local to Global: A Graph RAG Approach to Query-Focused Summarization
- https://www.youtube.com/watch?v=jCjyaQL-7mA&t=137s
- https://www.microsoft.com/en-us/research/blog/graphrag-unlocking-llm-discovery-on-narrative-private-data/
- https://www.microsoft.com/en-us/research/blog/graphrag-auto-tuning-provides-rapid-adaptation-to-new-domains/
- https://microsoft.github.io/graphrag/
- https://github.com/microsoft/graphrag/blob/main/RAI_TRANSPARENCY.md#what-is-graphrag
- https://github.com/hiryamada/graphrag-overview/tree/main
'📓 Papers' 카테고리의 다른 글
Attention is All You Need: Transformer와 LLM의 발전 양상 (0) | 2024.10.22 |
---|---|
Learning Single Camera Depth Estimation using Dual-Pixels (0) | 2021.10.03 |
HITNet: Hierarchical Iterative Tile Refinement Network for Real-time Stereo Matching (0) | 2021.10.03 |
SIMPLE ONLINE AND REALTIME TRACKING (0) | 2021.10.03 |
YOLOv4: Optimal Speed and Accuracy of Object Detection (0) | 2021.10.03 |